- October 18, 2021
- Posted by: Techsol Life Sciences
- Category: MedTech
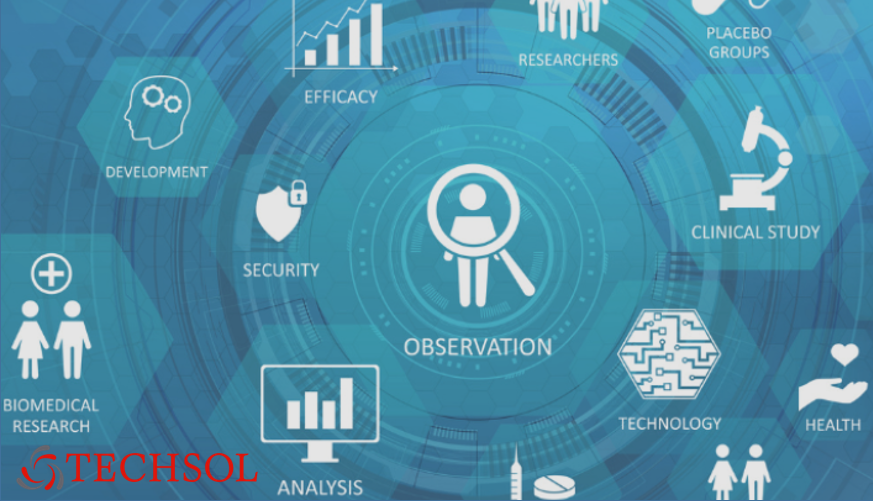
What drives the growing complexity in medical device clinical trials?
The medical devices industry has revolutionized medicine with significant advances in both detection and treatment of diseases. Clinical trials for medical device regulatory approval are designed to produce valid scientific evidence of safety and effectiveness for the intended uses. Moreover, sponsors must consider designing trials to demonstrate evidence required for payers, clinicians, and other important stakeholders. Typically, a core data set for a trial includes endpoints and data supporting regulatory approval, reimbursement, and other critical objectives.
There are rigorous regulations formulated by the U.S. Food and Drug Administration (FDA) and EU’s Medical Device (MDR) for class IIb and class III medical devices (i.e. medium and high-risk medical devices such as implantable medical devices or in vitro diagnostic devices- IVDR) [1] Regulators and payers are demanding more clinical evidence by device manufacturers to reflect the benefit-risk profile of the device. They need more crucial clinical evaluation before market approval and to substantiate product value claims. Clinical evidence of medical devices is critical not only for displaying the safety and efficacy of the device but also for notifying clinicians and patients about the ideal use of the device in the marketed clinical setting. This appeal for clinical evidence from various stakeholders is forcing medical device companies and sponsors to amass more “defensive clinical data” on their products than ever before. Companies are responding to this pressure by conducting extensive clinical trials, focus group studies, and responding in real-time by making changes to the software applications of their medical devices.
Here are 4 essential best practices for conducting clinical trials on medical devices.
Contact us by submitting a business inquiry online. We will get back to you very soon.
1. Blinding – MedTech Clinical Trials Bias And How To Avoid Them
Blinding is an essential part of a clinical trial as it diminishes measurement bias associated with the observer’s, doctor’s, or patient’s preconception. For ethical reasons, blinding is difficult to perform in randomized clinical trials of medical devices compared to therapeutic randomized clinical trials. Despite the practical difficulties with therapeutic procedures, some trials have been successfully conducted with complete blinding, involving an identical placebo. Blinding is complete when the interference is simulated in the control group with no contact between the surgeon and the team responsible for the patient’s follow-up.
Medical device companies need to ensure that when blinding is not workable, a blind assessment of the outcome should be planned with experienced staff as outcome assessors. They must also provide appropriate reasons for not blinding and discuss the limitations when reporting the results. In recent times, regulatory agencies have highlighted the use of creative methods to blind individuals in the trials.
Any novel blinding technique must ensure that it should have 3 basic qualities:
- Effective concealing of the group allocation
- No disruption in the ability to accurately assess outcomes
- Approval by the individuals that will be assessing outcomes
Regardless of careful contemplation of methods to blind individuals in medical device clinical trials, conditions will invariably arise when a certain group of individuals cannot ethically be blinded. MedTech companies can incorporate other alternative strategies to minimize bias when blinding is not possible such as “partial blinding”. This involves partial information being given to the participants, who may not be informed of standard treatment, e.g. the hypothesis that one treatment is superior to other, or of the randomization outcome for patients under a modified trial design. In these situations, patients must be advised on scientific grounds that, they will not be informed of the study’s specific objective but will have access to all the information at the end of the study.
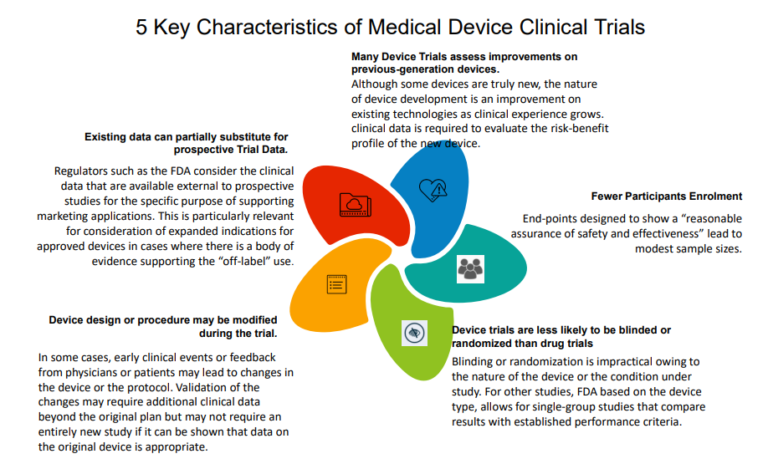
2. Sample Size Calculation Errors and How to Avoid It
Designing and organizing a clinical trial for a medical device is risky and expensive and to avoid the depletion of resources and time, it is essential to get the design right. The sample size is a critical component of any clinical trial. A trial with a small research group is more prone to inconsistency and carries a substantial risk of failing to demonstrate the effectiveness of a given intervention. There are specific recurrent errors that have been observed in trial records and protocols which can seriously compromise the scientific validity of the trial and therefore the regulatory approval.
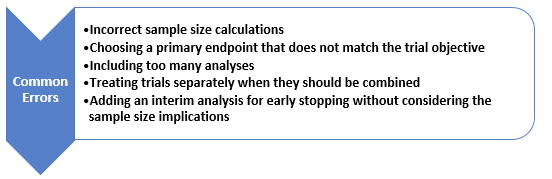
Figure 1. Common errors in sample size calculation
- Incorrect sample size calculations
The sample size is influenced by the interaction of prior knowledge of the device with the objective and number of subjects available. Generally, the more subjects, the more reliable the reported result will be; but at the same time more subjects equal more cost.
The sample size helps in prospectively estimating the number of subjects needed to provide the desired result. However, estimating sample size is complex as there is very limited data available about how the device will perform. If the trial gives erroneous results, any claim made has no validity and consequently leads to wastage of time and money.
- Using Interim analysis without considering the sample size
An interim analysis is helpful to identify if the trial can be stopped early by establishing that the primary endpoint has been achieved. This analysis to check for an early stop increases the sample size required for the study. Hence it is recommended to plan and document before the study starts, to avoid the risk of increasing the chance of a false positive study conclusion. Such conclusions could be scrutinized by regulators and are likely to result in rejection of the trial result.
- Collecting too much (or too little) data
Data collection and analysis is an expensive process and so it is important to consider what data is needed and its uses. Too much data can lead to difficulty in analyzing and compromises the quality of the important data, however, too little can compromise the entire study itself.
Example:
For example, changes in medication use can be recorded but is very hard to relate to an outcome, e.g. does 20mg paracetamol provide more or less pain relief than 60mg ibuprofen with a non-steroidal?
- Including too many analyses
Organizing too many analyses could lead to ambiguous and incorrect inferences. Secondary endpoints and subgroup analyses can quickly mount up unless there is a clear focus on what is being deduced.
3. Selecting the Right Primary and Secondary End Points
An endpoint is a single trial outcome value that is measured for each subject. To address the trial objective, the individual subject endpoint data must be summarised. This may be a simple or more complex statistical process. [2]
Thus, inadequately defining the endpoint may result in failing to address the objective, and therefore falls short to satisfy the regulators.
Primary Endpoint
The trial design should evaluate the safety and effectiveness of the device in a given population under study. In general, it is divided into one or more primary “safety” and “effectiveness” endpoints. A study would be considered successful if both the safety and effectiveness endpoints are met [3].
The clinical trial protocol should evidently and prospectively detail
- Comprehensive methods for obtaining endpoint data
- Descriptions for what will be counted as a primary event in the analysis
- Circumstances in which patient data will be excluded
- How missing data will be handled
- How the impact of covariates will be assessed[3]
To determine the primary endpoint a minimum number of patients and duration of follow-up may be required depending on understanding the safety and effectiveness of the device.
Secondary Endpoints
Secondary endpoints are used to evaluate additional claims where they provide further insights into the device’s effects and mechanisms of action. Submission Elements, Pivotal Investigational device exemption (IDEs), feasibility study provide enough detail to avoid ambiguity once the trial has started. Secondary endpoints are mostly used to support claims if the primary endpoints are successful.
4. Choosing Right Exploratory and Inferential Statistical Methods for MedTech clinical trials
Statistical Approaches to Analysis of Clinical Trials
An indispensable element to a well-designed clinical trial is its appropriate statistical analysis. Presuming that a clinical trial will provide data that could reveal differences between two or more mediations, statistical analyses are used to determine whether such differences are real or are due to chance. Data analysis for clinical trials is important to make a clear distinction between preliminary evidence and confirmatory analysis. When the sample population size is small, it is essential to gather considerable preliminary evidence on related subjects before the trial is conducted to define the size needed to determine a critical effect. For example, a trial with eight participants may have adequate statistical power, expressing the probability of rejecting the null hypothesis when the hypothesis is false.
Thus, testing a null hypothesis could be challenging for a small clinical trial framework. It is therefore important to focus on evidence rather than to test a hypothesis. This is because a small clinical trial is less likely to be self-contained, providing all of the necessary indications to effectively test a particular hypothesis. A single large clinical trial is often inadequate to answer a biomedical research question, and it is even more unlikely that a single small clinical trial can do so. Thus, analyses of data must consider the limitations of the data at hand and their background in contrast with those of other related studies [4].
Since data analysis for small clinical trials unavoidably involves a number of assumptions, it is reasonable that several different statistical analyses be conducted. If these analyses give consistent results under different assumptions, one can be assured that the results are not due to unwarranted expectations [4].
References:
1. Joshi, S. (2020, June 1). Clinical Trials for Medical Devices: 5 Expert Tips. The Kolabtree Blog. https://www.kolabtree.com/blog/clinical-trials-for-medical-devices-5-expert-tips/
2. Yellowlees, I. (2021, June 12). Sample Size and Other Common Medical Device Trial Design Errors that Matter. Quantics Biostatistics. https://www.quantics.co.uk/blog/6-common-medical-device-trial-design-errors-that-matter/
3. [Image].Https://Www.Slideshare.Net/Medicres/Fda-2013-Clinical-Investigator-Training-Course-Issues-in-Clinical-Trials-Designs-for-Devices
4. NCBI Bookshelf. (2001). Retrieved 2021, from https://www.ncbi.nlm.nih.gov/books/NBK223333/